Quantum Machine Learning: The Future of AI Training?
- The Tech Platform
- Mar 16, 2024
- 5 min read
Imagine training AI on problems that are impossible for today's computers! That's the promise of Quantum Machine Learning (QML). It's a new way of training AI that uses the strange rules of quantum mechanics. While regular AI relies on regular computers, Quantum Machine Learning uses special quantum computers that can solve super complex problems. This article will explore how Quantum Machine Learning works and how it could revolutionize fields like medicine and materials science!
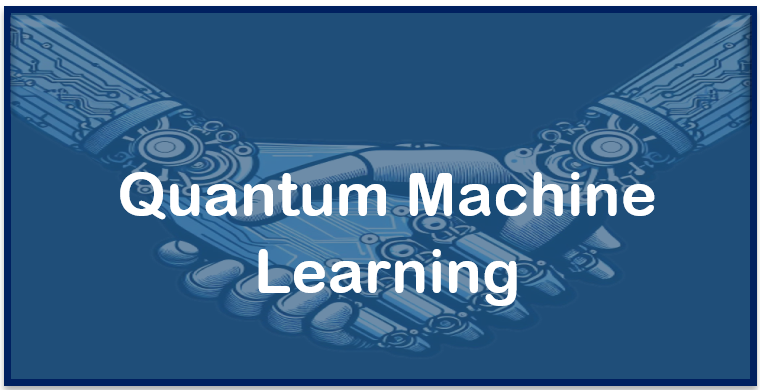
Quantum Machine Learning
Quantum Machine Learning (QML) is a field of artificial intelligence (AI) and quantum mechanics. It seeks to leverage quantum computers' unique computational power to revolutionize how AI models are trained and problems are tackled. Here's a deeper dive into the essence of QML:
Classical AI vs. Quantum AI:
Traditional AI relies on classical computers, which operate on bits - the fundamental unit of information represented as either 0 or 1. These computers excel at processing well-defined problems with clear rules. However, classical computers struggle with problems that involve massive search spaces, complex interactions, or inherent randomness.
Quantum Machine Learning steps in to address these limitations by harnessing the power of quantum mechanics. Quantum mechanics governs the behavior of matter and energy at the atomic and subatomic level, exhibiting phenomena that are counterintuitive to our everyday experiences.
These phenomena become the foundation for Quantum Machine Learning:
Qubits: The workhorses of quantum computers are qubits, the quantum equivalent of bits. Unlike bits, qubits can exist in a superposition state, meaning they can be 0, 1, or both simultaneously. This ability allows quantum computers to explore a vast number of possibilities concurrently, making them ideal for tackling problems with exponentially large search spaces.
Quantum Phenomena: Concepts like entanglement, where two qubits become linked regardless of their physical separation, further enhance the capabilities of Quantum Machine Learning algorithms. By leveraging these phenomena, QML can perform computations that are intractable for classical computers.
So, why is Quantum Machine Learning such a big deal for AI? Because it can tackle problems that are impossible for regular computers. This opens doors to incredible breakthroughs in fields like medicine and materials science, which we'll explore later. But for now, remember: Quantum Machine Learning uses qubits and entanglement to unlock the true potential of AI!
Quantum Machine Learning Algorithms
Regular AI relies on regular computer programs. Quantum Machine Learning (QML) is different! It uses special algorithms designed to work with the strange rules of quantum mechanics. These algorithms are like superpowers for AI, allowing it to tackle problems that are impossible for today's computers.
Let's look at some of the coolest ones:
Quantum Variational Eigensolvers (VQEs):
Imagine searching for the best needle in a haystack with millions of needles. That's the kind of challenge VQEs excel at. They tackle optimization problems where you need to find the absolute best solution from a massive number of possibilities. These problems are a nightmare for classical computers, but VQEs use the power of superposition to explore many solutions simultaneously, making them ideal for tasks like:
Drug discovery: Simulating complex molecular interactions to design new drugs faster and more efficiently.
Materials science: Finding materials with specific properties, like superconductors that operate at room temperature.
Quantum Support Vector Machines (QSVM):
Machine learning often involves tasks like image recognition or classifying data points. QSVM algorithms aim to bring quantum power to these tasks. By harnessing entanglement, QSVMs could potentially process information and learn patterns much faster than classical Support Vector Machines, leading to:
Improved image and speech recognition: Imagine AI that can identify objects in a cluttered scene with superhuman accuracy.
Enhanced financial modeling: QSVMs could analyze vast amounts of financial data to identify trends and make more accurate predictions.
Quantum Generative Adversarial Networks (QGANs):
These are still in their early stages, but QGANs hold immense potential for generating new data and pushing the boundaries of AI creativity. Imagine an AI that can design new materials with never-before-seen properties or create realistic simulations for training other AI systems.
Potential Breakthroughs with Quantum AI
Quantum Machine Learning (QML) isn't just about solving complex problems; it's about unlocking a new era of breakthroughs across various fields.
Let's explore how QML could revolutionize some key areas:
1. Drug Discovery: From Years to Days?
Developing new drugs is a slow and expensive process. It involves simulating how potential drug molecules interact with complex biological systems. By harnessing the power of quantum computers, QML algorithms like Quantum Variational Eigensolvers (VQEs) can simulate these interactions with much greater accuracy and speed.
Imagine going from years of testing to identifying promising drug candidates in a matter of days! This could lead to faster development of life-saving medications for diseases like cancer or Alzheimer's.
2. Materials Science: Designing the Impossible
Materials science is all about creating new materials with specific properties. Think lightweight yet super strong materials for airplanes or superconductors that operate at room temperature. However, simulating these materials at the atomic level is computationally expensive for classical computers.
Quantum Machine Learning algorithms can tackle this challenge. By performing complex simulations with unmatched accuracy, QML could pave the way for the design of entirely new materials with previously unimaginable properties, revolutionizing fields like engineering, energy, and construction.
3. Finance: Seeing the Market in a Quantum Light
The financial world thrives on complex models that analyze vast amounts of data to predict market trends and manage risk. However these models are often limited by computational power. Enter Quantum Machine Learning algorithms like Quantum Support Vector Machines (QSVM). These algorithms could process financial data much faster and identify hidden patterns that classical machines miss. This could lead to more accurate risk assessments, better investment strategies, and a more stable financial landscape.
These are just a few examples, and the potential applications of Quantum Machine Learning are vast. From optimizing logistics to personalizing medicine, QML transforms industries and improves our lives in ways we can only begin to imagine.
Challenges and Future Directions
While the potential of Quantum Machine Learning (QML) is undeniable, there are hurdles to overcome before it reaches its full potential. Here's a glimpse into the current limitations and ongoing efforts to address them:
Challenges:
Limited Qubit Coherence Times: Qubits, the building blocks of quantum computers, are fragile and prone to errors. They can lose their quantum information (their superposition state) after a short period, hindering the accuracy of computations.
Specialized Algorithms: Most classical machine learning algorithms need significant adaptation to work effectively on quantum computers. Developing entirely new algorithms specifically designed for QML architectures is an ongoing challenge.
Scalability: Building and maintaining large-scale quantum computers remains a complex feat. Currently, the number of qubits in functional quantum computers is limited, restricting the complexity of problems they can tackle.
Future Directions:
Researchers are actively working on overcoming these limitations:
Error Correction Techniques: Several approaches are being explored to improve qubit coherence times and minimize errors. These include quantum error correction codes and techniques for actively mitigating errors during computations.
Hybrid Quantum-Classical Algorithms: Marrying the strengths of both worlds is another promising approach. Here, classical computers handle the tasks they excel at, while quantum computers tackle specific sub-problems where they offer a clear advantage.
Advancements in Hardware: Significant research is dedicated to building more robust and scalable quantum computers. This includes exploring new materials and architectures that can house a larger number of stable qubits.
Overcoming these challenges is an ongoing process, but the potential rewards justify the effort. As these issues are addressed, we can expect a significant leap in QML capabilities. The future holds immense promise for a new era of AI powered by quantum mechanics, pushing the boundaries of scientific discovery and technological innovation.
Conclusion
Quantum Machine Learning (QML) is like giving AI a superpower! It uses the strangeness of quantum mechanics to tackle problems that are impossible for today's computers. This could lead to amazing breakthroughs in medicine, materials science, and more!
There are still challenges to overcome, but scientists are working hard to make Quantum Machine Learning even more powerful. The future of AI looks bright, thanks to the exciting potential of Quantum Machine Learning!
Comments