
Information extraction is the process of extracting information from unstructured textual sources to enable finding entities as well as classifying and storing them in a database. Semantically enhanced information extraction (also known as semantic annotation) couples those entities with their semantic descriptions and connections from a knowledge graph. By adding metadata to the extracted concepts, this technology solves many challenges in enterprise content management and knowledge discovery.
How Does Information Extraction Work?
There are many subtleties and complex techniques involved in the process of information extraction, but a good start for a beginner is to remember.

To elaborate a bit on this minimalist way of describing information extraction, the process involves transforming an unstructured text or a collection of texts into sets of facts (i.e., formal, machine-readable statements of the type “Bukowski is the author of Post Office“) that are further populated (filled) in a database (like an American Literature database).
Typically, for structured information to be extracted from unstructured texts, the following main subtasks are involved:
Pre-processing of the text – this is where the text is prepared for processing with the help of computational linguistics tools such as tokenization, sentence splitting, morphological analysis, etc.
Finding and classifying concepts – this is where mentions of people, things, locations, events and other pre-specified types of concepts are detected and classified.
Connecting the concepts – this is the task of identifying relationships between the extracted concepts.
Unifying – this subtask is about presenting the extracted data into a standard form.
Getting rid of the noise – this subtask involves eliminating duplicate data.
Enriching your knowledge base – this is where the extracted knowledge is ingested in your database for further use.
Information extraction can be entirely automated or performed with the help of human input.
Typically, the best information extraction solutions are a combination of automated methods and human processing.
Typical Information Extraction Applications
Information extraction can be applied to a wide range of textual sources: from emails and Web pages to reports, presentations, legal documents and scientific papers. The technology successfully solves challenges related to content management and knowledge discovery in the areas of:
Business intelligence (for enabling analysts to gather structured information from multiple sources);
Financial investigation (for analysis and discovery of hidden relationships);
Scientific research (for automated references discovery or relevant papers suggestion);
Media monitoring (for mentions of companies, brands, people);
Healthcare records management (for structuring and summarizing patients records);
Pharma research (for drug discovery, adverse effects discovery and clinical trials automated analysis).
Steps involved in extracting information from Raw Text
Although textual data is abundantly available, the entanglement of natural language makes it particularly difficult to extract useful information from them. However, no matter how complex the Information Extraction task, there are some common steps that form the pipeline of almost all IE systems.

Tokenization
Tokenization is a part of Lexical Processing that is usually performed as a preliminary task in NLP applications. Tokenization involves splitting text documents into semantically meaningful units such as sentences and words (tokens).
Generally, sentence tokenization is done by splitting the text at the sentence-endings(‘.’) and then the word tokenization by splitting the sentence at the Blank Space. Nevertheless, Sophisticated methods are used for tokenizing more complex text structures, such as, the words that often go together like “Los Angeles”, which are sometimes known as collocations.
Part-of-Speech Tagging
Part-of-Speech (PoS) Tagging is the first level of Syntactic Processing that tags the word to the role it plays in a sentence. Some general forms of pos tags are nouns, verbs, pronouns, adjectives, adverbs, prepositions, interjection, conjunction, etc. Below is the example of Pos Tagging.

The set of standard PoS tags used in the NLTK library by default is included in the reference section. In Fact, it’s not a straightforward task to tag a word, for instance, in the following sentence “the song is a big hit”, the word “hit” is a Noun, whereas, in the sentence “he hit me”, “hit” is a Verb. Handling such ambiguity needs more Advanced techniques that deserve a separate blog.
Named-entity Recognition and Relation Extraction
A crucial component in IE systems is Named Entity Recognition (NER). Named-entity recognition is the problem of identifying and classifying entities into categories such as the names of people, locations, organizations, the expressions of quantities, times, measurements, monetary values, and so on. In general terms, entities refer to names of people, organizations (e.g. Jet Airways, American Airlines), places/cities (Bengaluru, Boston), etc.

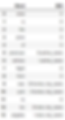
In entity recognition, every token is tagged with an IOB label and then nearby tokens are combined together based on their labels. IOB labels (I-inside, O-out, B-beginning) are something similar to PoS tagging but it includes domain-specific custom labels. For instance, for the user request, ‘What is the price of American Airlines flight from New York to Los Angeles’ the tagged IOB labels are on the left.
Once we find the PoS tags and the IOB labels, it is a task of mapping the relationships between entities.
NLP (Natural Language Processing) Techniques for Extracting Information
Let’s explore 5 common techniques used for extracting information from the above text.
1. Named Entity Recognition
The most basic and useful technique in NLP is extracting the entities in the text. It highlights the fundamental concepts and references in the text. Named entity recognition (NER) identifies entities such as people, locations, organizations, dates, etc. from the text.
NER output for the sample text will typically be:
Person: Lucas Hayes, Ethan Gray, Nora Diaz, Sofia Parker, John
Location: Brooklyn, Manhattan, United States
Date: Last month, 2015
Organization: Rocketz
NER is generally based on grammar rules and supervised models. However, there are NER platforms such as open NLP that have pre-trained and built-in NER models.
2. Sentiment Analysis
The most widely used technique in NLP is sentiment analysis. Sentiment analysis is most useful in cases such as customer surveys, reviews and social media comments where people express their opinions and feedback. The simplest output of sentiment analysis is a 3-point scale: positive/negative/neutral. In more complex cases the output can be a numeric score that can be bucketed into as many categories as required.
In the case of our text snippet, the customer clearly expresses different sentiments in various parts of the text. Because of this, the output is not very useful. Instead, we can find the sentiment of each sentence and separate out the negative and positive parts of the review. Sentiment score can also help us pick out the most negative and positive parts of the review:
Most negative comment: The call center guys are extremely rude and totally ignorant.
Sentiment Score: -1.233288
Most positive comment: The premium is reasonable compared to the other insurance companies in the United States.
Sentiment Score: 0.2672612
Sentiment Analysis can be done using supervised as well as unsupervised techniques. The most popular supervised model used for sentiment analysis is naïve Bayes. It requires a training corpus with sentiment labels, upon which a model is trained which is then used to identify the sentiment. Naive Bayes is not the only tool out there - different machine learning techniques like random forest or gradient boosting can also be used.
The unsupervised techniques also known as the lexicon-based methods require a corpus of words with their associated sentiment and polarity. The sentiment score of the sentence is calculated using the polarities of the words in the sentence.
3. Text Summarization
As the name suggests, there are techniques in NLP that help summarize large chunks of text. Text summarization is mainly used in cases such as news articles and research articles.
Two broad approaches to text summarization are extraction and abstraction. Extraction methods create a summary by extracting parts from the text. Abstraction methods create summary by generating fresh text that conveys the crux of the original text. There are various algorithms that can be used for text summarization like LexRank, TextRank, and Latent Semantic Analysis. To take the example of LexRank, this algorithm ranks the sentences using similarity between them. A sentence is ranked higher when it is similar to more sentences, and these sentences are in turn similar to other sentences.
Using LexRank, the sample text is summarized as: I have to call the call center multiple times before I get a decent reply. The premium is reasonable compared to the other insurance companies in the United States.
4. Aspect Mining
Aspect mining identifies the different aspects in the text. When used in conjunction with sentiment analysis, it extracts complete information from the text. One of the easiest methods of aspect mining is using part-of-speech tagging.
When aspect mining along with sentiment analysis is used on the sample text, the output conveys the complete intent of the text:
Aspects & Sentiments:
Customer service – negative
Call center – negative
Agent – negative
Pricing/Premium – positive
5. Topic Modeling
Topic modeling is one of the more complicated methods to identify natural topics in the text. A prime advantage of topic modeling is that it is an unsupervised technique. Model training and a labeled training dataset are not required.
There are quite a few algorithms for topic modeling:
Latent Semantic Analysis (LSA)
Probabilistic Latent Semantic Analysis (PLSA)
Latent Dirichlet Allocation (LDA)
Correlated Topic Model (CTM).
One of the most popular methods is latent Dirichlet allocation. The premise of LDA is that each text document comprises of several topics and each topic comprises of several words. The input required by LDA is merely the text documents and the expected number of topics.
Using the sample text and assuming two inherent topics, the topic modeling output will identify the common words across both topics. For our example, the main theme for the first topic 1 includes words like call, center, and service. The main theme in topic 2 are words like premium, reasonable and price. This implies that topic 1 corresponds to customer service and topic two corresponds to pricing. The diagram below shows the results in detail.
Conclusion
These are just a few techniques of natural language processing. Once the information is extracted from unstructured text using these methods, it can be directly consumed or used in clustering exercises and machine learning models to enhance their accuracy and performance.
The Tech Platform