Distributed Systems and Asynchronous I/O
- The Tech Platform
- Apr 12, 2021
- 10 min read
How different forms of handling I/O affect the performance, availability, and fault-tolerance of network applications.
Dealing with distributed systems is quite a difficult job. There could be numerous components developed by different teams over long periods. Human mistakes, such as shipping bugs or incorrectly configured parameters, happen routinely in large systems, no matter how excellent the engineering practices are. Hardware faults also regularly occur in large fleets. On top of that, unpredictable workloads and permutations of all possible states and conditions make it virtually impossible to foresee what might go wrong.
That’s why it’s essential to limit the blast radius to avoid cascading failures and amplified outages. Also, it is no less critical to strive for simplicity to make systems easier to reason about.
There are multiple aspects and techniques for dealing with distributed systems, and I will cover some of them in the future. While this domain has a depth of knowledge to explore, this post will focus on basics, comparing different forms of handling I/O and how they affect network applications’ availability, performance, and fault-tolerance.
Non-blocking I/O is a mature and widely known concept. Still, there are plenty of services and SDKs built using blocking I/O. Some engineers assume that it’s just a matter of the number of threads that can compensate for the difference. And because blocking/synchronous programs are much easier to write, read and maintain, dealing with async I/O is not worth it.
I’ll try to use simple analogies and examples to show why the difference is more than just the number of threads. Also, I’ll touch on the topic of writing asynchronous code. And because: “I hear and I forget, I see and I remember, I do and I understand.”
I created a modeling tool for visualization and empirical verification of the below-presented points, so you also can play around with different scenarios if you’d like to explore the topic.
Let me start with some basic concepts.
Thread-safety and concurrency in intuitive terms
Balancing thread-safety and concurrency is non-trivial. For one, we want to avoid data races, specifically, modifying the same data with more than one thread simultaneously, which may lead to data corruption and inconsistency. Conversely, we want our applications to run fast and to minimize thread contention. Thread-safety and concurrency are somewhat at odds, and if thread-safety is done wrong, it may result in low concurrency.
I find the road traffic analogy very helpful to reason intuitively about thread-safety and concurrency. Let’s say we have tasks, which are vehicles, and threads, which are roads. Then, thread-safety is similar to avoiding collisions, while concurrency is about the road system’s bandwidth.
There are different ways to ensure the absence of collisions, each with its own pros and cons.
Immutable data
If data is never changed, it is safe to share it between threads. This is the best combination of thread-safety and concurrency, but it has limited application. It is like road signs: there is no limit on how many people can simultaneously look at them, but no one can interact with them. It is also suitable for rarely changing snapshots and relaxed consistency requirements.
Thread-local data
Each thread has its own isolated replica, which is similar to “each car has its own lane.” It is like single lane roads that never cross — safe but not always useful. This approach is performant but might be expensive and often leads to memory over-subscription.
Compare-and-swap/atomic operations
Atomic operations guarantee data consistency without locking. I consider it a 4-way stop sign where each thread needs to stop and check its surroundings before crossing an intersection. This approach works well for sharing pointers, flags, and counters between threads. It may inhibit performance if misused. You can learn more here: Chapter “8.2.2. Memory ordering.”
Locks/mutexes
Similar to traffic lights, they enable only a single thread to access a resource at a given point in time. In case of concurrent access, all other threads wait, sometimes for a freight train to pass. But it might be better to have occasional traffic lights than too many stop signs.
So, if your road system (service) has too many traffic lights (mutexes/locks) and stop signs (volatile/atomic/memory barriers), the traffic would move slowly.
For instance, let’s benchmark incrementing a counter shared between multiple threads (using Rust and Criterion):
fn benchmark_batched_increment(
repetitions: usize,
mut increment: usize,
counter: &AtomicUsize)
{
let mut batch =0;
for _ in 0..repetitions {
// avoiding compiler optimizations
// E.g. go to https:
//rust.godbolt.org/z/7he65h
// and try to comment the line #4
increment = increment ^1;
batch += increment;
}
counter.fetch_add(batch, Ordering::Relaxed);
}
fn benchmark_atomic_increment(
repetitions: usize,
mut increment: usize,
counter: &AtomicUsize)
{
for _ in0..repetitions {
increment = increment ^1;
counter.fetch_add(increment, Ordering::Relaxed);
}
}
fn benchmark_mutex_increment(
repetitions: usize,
mut increment: usize,
counter_mutex: &Mutex<usize>,
) {
for _ in 0..repetitions {
increment = increment ^1;
let mut lock = counter_mutex.lock()
.expect("Never fails in this bench");
*lock += increment;
}
}
Using the most relaxed Atomic guarantees
Which gives the following results on Intel(R) Xeon(R) CPU @ 2.30GHz:
Increment Batched time: 0.0646 us
Increment Atomic time: 7.3110 us
Increment Mutex time: 23.123 us
Batched 72232381 operations, 1.000000
Atomic 605543 operations, 0.008383
Mutex 174110 operations, 0.002410
As you can see, naïve Atomic increment is ~125 times slower than batching increments in the local memory and then updating a shared counter. Using a Mutex is slower ~400 times. In a multi-threaded environment, these differences will be even more considerable because of thread contention. Please note, the difference may vary for different scenarios.
Simply put, it is like driving on a freeway vs. stopping at every intersection vs. having traffic-lights at every corner.
We want to organize our workflow so that, most of the time, each thread doesn’t have to stop and either has exclusive access to its data or shared access to read-only data. So we drive on a freeway with occasional but quick merges to avoid congestion as much as we can.
Coarse-grained locking
Once, I joined a project which had the following pattern all over the place:
synchronized (outputStreamLock) {
outputStream.write(event);
}
Such an approach might be okay if your app is meant to serve a few dozens of requests per second. It was not the case, so the team had to deal with a massive fleet. Therefore it was time to redesign the I/O ownership model. But what is wrong here? First of all, outputStream doesn’t have a single owning thread. Multiple threads can attempt to write concurrently to the same stream:

As you can see from the diagram, we used two threads where a single one was sufficient. In some cases, it might be multiple threads (in the worst case, all of your threads) waiting for each other and wasting expensive resources. Fine-grained locking would work much better:
BlockingQueue<Event> eventQueue = new LinkedBlockingDeque<>();
...
// worker threads
eventQueue.offer(event);
...// The thread owning `outputStream`:
while (!done) {
outputStream.write(eventQueue.poll());
}

Now worker threads do not wait for expensive writes to complete because they send data to the thread owning the output stream. Yes, they still might block each other, but those pauses are brief, especially compared to I/O operations, and there are multiple techniques to reduce contention.
In other words, the former approach was just a very expensive queue, which may lead to exhaustion of your thread pool under certain conditions, such as a network slowdown or an unresponsive dependency. Getting back to the traffic metaphor, this is what the first approach may look like under heavy load:
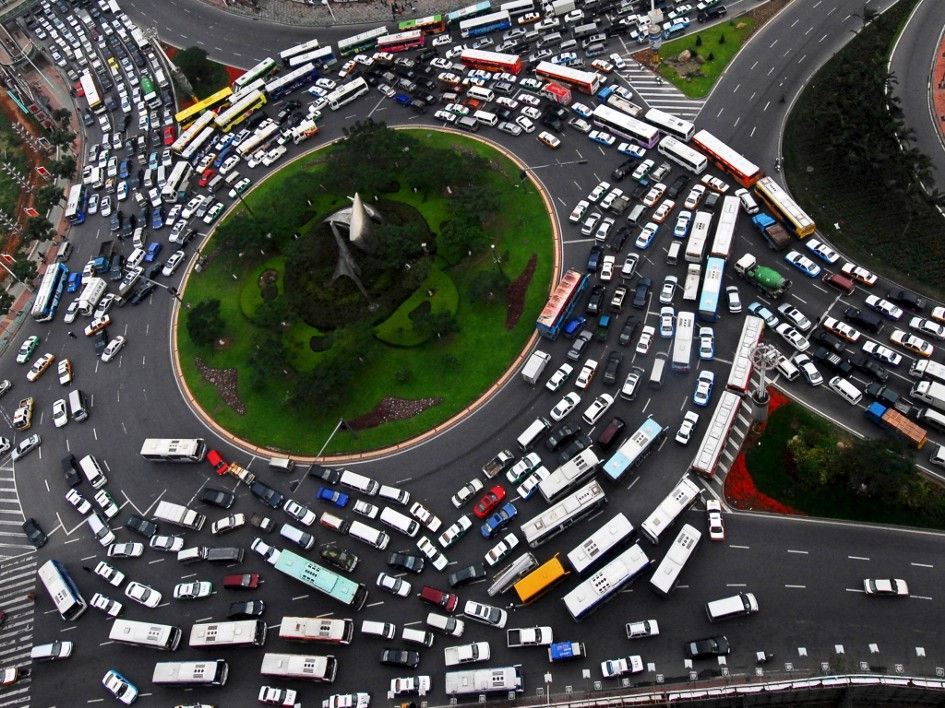
Coarse-grained locking. Note the empty roads (idle threads).
and this is how the second one can be seen:
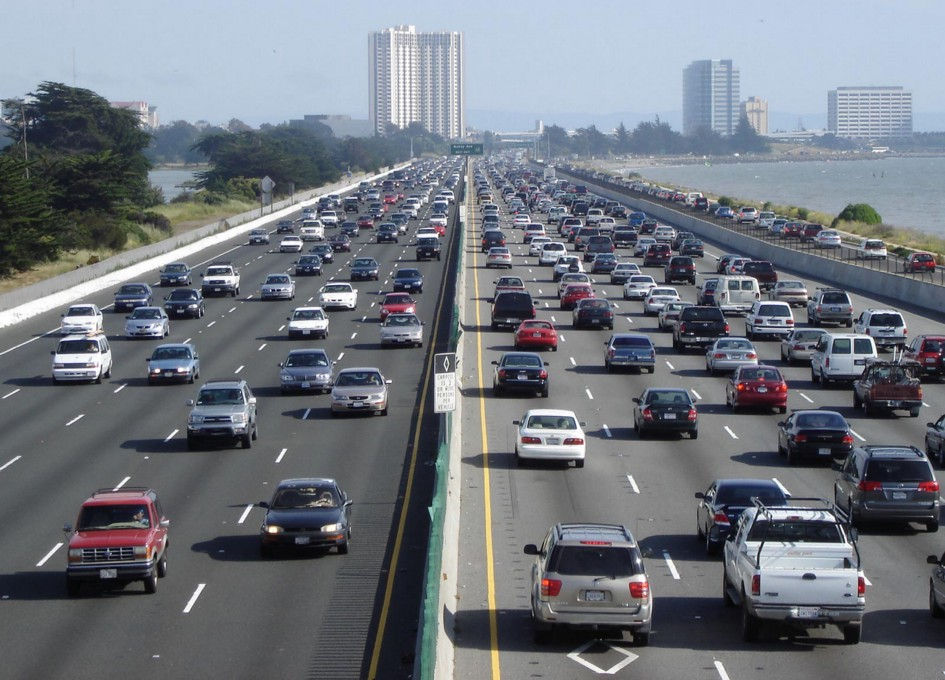
Fine-grained locking.
Comparing blocking and non-blocking I/O
Okay, that was an example of quite a sub-optimal way of balancing thread-safety and concurrency. What about something like this:
ExecutorService service = Executors.newFixedThreadPool(MANY);
...
service.execute(() -> {
// RPC = Remote Procedure Call
Result r = dependency.makeRpcCall(parameters); // blocking
process(r); // let's assume it's instant
});
So we have presumably a sufficiently large number of threads to make remote calls, and process the results. For the sake of simplicity, we can assume we don’t have to deal with thousands of long-running connections (as each thread may handle only a single connection at a time).
We can compare it with asynchronous processing and see if there is any difference in performance and fault-tolerance.
Let’s start with the following scenario:
Request rate = 1000 RPS (requests per second).
Request latency = 20ms (with zero variance).
10 upstream endpoints handle our RPC calls (with round-robin selection).
To compare synchronous and asynchronous approaches, we’ll use the following metrics:
Average throughput in RPS and standard deviation.
Latency overhead — i.e., the latency added by the service on top of the RPC call, measured in milliseconds.
Latency overhead distribution — using percentiles. E.g., p99 — the value below which 99% of latency observations fall.
Let’s run the modeling tool, which also renders throughput and latency distribution diagrams (all tests below have been performed on 4 cores, Intel(R) Xeon(R) CPU @ 2.30GHz, GCC version 8.3.0, Debian 4.19.152–1 (2020–10–18)):
concurrency-demo-benchmarks \
--name sync_1k_20ms \
--rate 1000 \
--num_req 10000 \
--latency "20ms*10" \
sync --threads 50
and to model async I/O processing:
concurrency-demo-benchmarks \
--name async_1k_20ms \
--rate 1000 \
--num_req 10000 \
--latency "20ms*10" \
async
For both cases, the throughput is identical:
Sync: Avg rate: 1000.000, StdDev: 0.000
Async: Avg rate: 1000.000, StdDev: 0.000
In this scenario, the latency overhead is low for both and rather driven by sleep/delay granularity (usually 1ms) than processing overhead. So far, so good. What about handling traffic spikes? Let’s dial up the rate to 2,000 RPS:
Sync: Avg rate: 1995.857, StdDev: 10.148
Async: Avg rate: 2000.000, StdDev: 0.000
Interesting, it seems that the sync case has more thread contention while accessing the task queue (which is shared between threads). Here and everywhere below, sync is on the left, async is on the right:
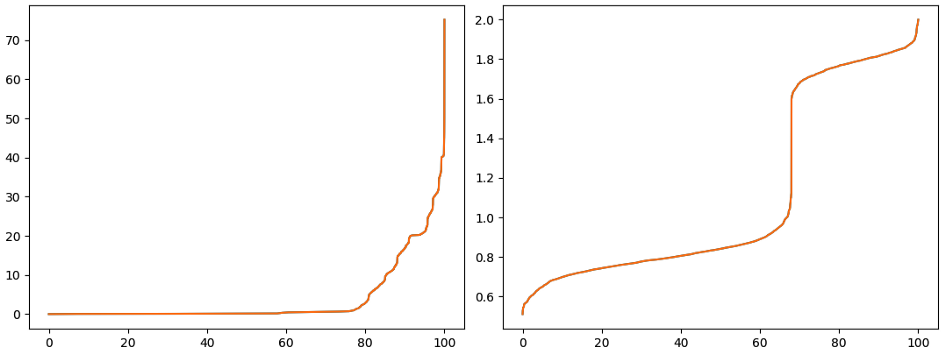
X-axis — percentiles, Y-axis — overhead in milliseconds. Please note the Y-axis scale difference.
The tail latency is much worse for the sync case for higher request rates. Partitioning the task queue may alleviate this, but it would require balancing tasks between partitions, which is just another non-trivial problem.
Now, let’s explore what might happen if one of the upstream endpoints becomes unavailable, so remote calls start to time out (timeout = 30s):

10% of the nodes went down.
All threads will almost instantly block, and our service is 100% unavailable just because 10% of our dependency went down. But this won’t affect the asynchronous case at all. Let’s run the simulation:
concurrency-demo-benchmarks \
--name sync_1k_30s \
--rate 1000 \
--num_req 10000 \
--latency "20ms*9,30s" \
sync --threads 50
concurrency-demo-benchmarks \
--name async_1k_30s \
--rate 1000 \
--num_req 10000 \
--latency "20ms*9,30s" \
async
The difference in throughput is dramatic:
Sync: Avg rate: 14.670, StdDev: 53.997
Async: Avg rate: 900.000, StdDev: 0.000
While the asynchronous app can maintain 900 RPS (as only 90% of requests complete successfully), the synchronous’ throughput starts to oscillate down to almost zero because a large portion of threads is just waiting:

Also, note that it took ~100 times longer to complete all requests. X-axis — a timeline in seconds, Y-axis RPS.
The overhead latency quickly goes to hundreds of seconds for the synchronous case, while for the asynchronous one, the overhead is quite manageable with p100 ~2ms:
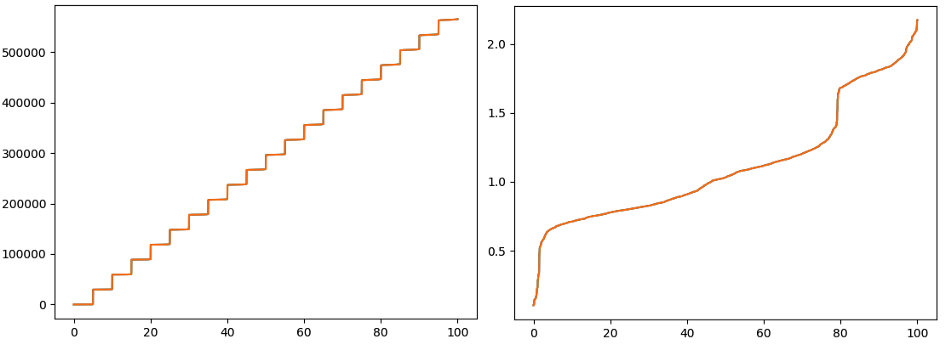
Blocking I/O (on the left) is really poor handling upstream timeouts. X-axis — overhead percentiles, Y-axis milliseconds.
Let’s do “slo-mo” by magnifying the latency to 200ms (and scaling the number of threads accordingly) to see how exactly the service’s latency degrades:
concurrency-demo-benchmarks \
--name sync_slo_mo_1k_30s \
--rate 1000 \
--num_req 10000 \
--latency "200ms*9,30s" \
sync --threads 500
The tail latency shoots up pretty quickly, but even p50 (i.e., the median latency) catches up very soon:

X-axis — a timeline in seconds. Y-axis — latency overhead. Blue — p50, Orange — p90, Green — p99 in ms.
Of course, we can add some engineering to avoid sending requests to unhealthy nodes, tracking the number of pending requests, or using shuffle-sharding, so each service node sends requests only to a subset of upstream nodes to reduce blast radius, etc. However, all of those would still leave room for hiccups, as such detection won’t be instant. Also, it would add more complexity, which, in general, means more states, more bugs, more cognitive load, and heavier operations.
BTW, this scenario can cascade and lead to a situation when a relatively small subset of nodes sends ripples across the entire service, affecting its availability:
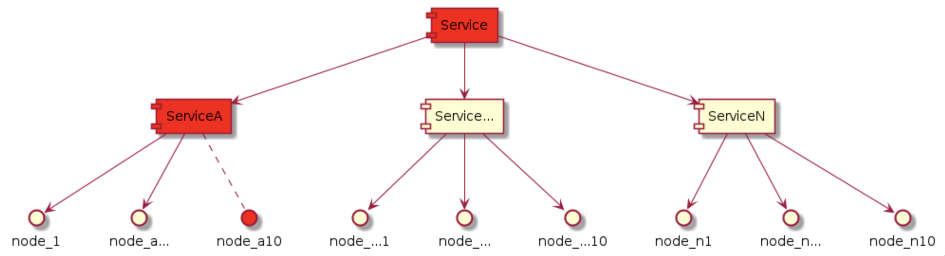
Similar to a single stalled car blocking freeway miles around, if the timing is bad.
Okay, let’s see what happens if our dependencies slow down, and the RPC latency goes up from 20ms to 60ms. Would it affect the bandwidth? Let’s do some math:
Bandwidth(latency) = number_of_threads/latency
Bandwidth(20ms) = 50/0.02 = 2,500 rps
Bandwidth(60ms) = 50/0.06 = ~833 rps
Oops, now we are under the 1,000 RPS, and each second we have ~167 requests building up in the request queue (and eventually timeout). For the asynchronous case, it doesn’t make any difference.
Let’s verify this math empirically:
concurrency-demo-benchmarks \
--name sync_1k_60ms \
--rate 1000 \
--num_req 10000 \
--latency "60ms*10" \
sync -t 50
As expected, the throughput decreased, just below the theoretical bandwidth limit:
Sync: Avg rate: 832.444, StdDev: 3.624
Async: Avg rate: 1000.000, StdDev: 0.000

The blocking I/O throughput is affected. X-axis — a timeline in seconds, Y-axis RPS.
The latency overhead quickly builds up to seconds, while for the asynchronous case, it didn’t change:

The queuing of requests moves latency to seconds. X-axis — overhead percentiles, Y-axis
milliseconds.
Sure, spinning up more threads would help. But the memory cost is not negligible, and you may end up dealing with memory-hungry applications and underutilized hardware as a result. At some point, you’ll hit the law of diminishing returns, wasting too much memory and CPU:
# Limiting the thread arena size
# Learn more: https://sourceware.org/glibc/wiki/MallocInternals#Arenas_and_Heaps
~$ export MALLOC_ARENA_MAX=1
# A command to see the memory consumption
~$ ps -ef | grep concurrency-demo-benchmarks | grep -v grep |
awk {'print $2'} | xargs pmap | tail -n 1
# running in the async mode
total 13748K # ~0.014 GB
# sync mode, 1 thread
total 15812K # ~0.016 GB
# sync mode, 10 threads
total 34388K # ~0.034 GB
# sync mode, 100 threads
total 220280K # ~0.22 GB
# sync mode, 1000 threads
total 2079200K # ~2.1 GB
# sync mode, 10,000 threads
total 20668660K # ~20.7 GB
As you can see, it takes about 2Mb per every thread (may vary by platform/settings). So increasing bandwidth by adding more threads might be costly.
Last but not least, let’s explore the system bandwidth. For the async case, we can easily dial up the RPS to 10,000, and it affects neither the latency nor the throughput stability, with just ~0.03 GB of memory:
concurrency-demo-benchmarks \
--name async_10k_20ms \
--rate 10000 \
--num_req 100000 \
--latency "20ms*10" \
async
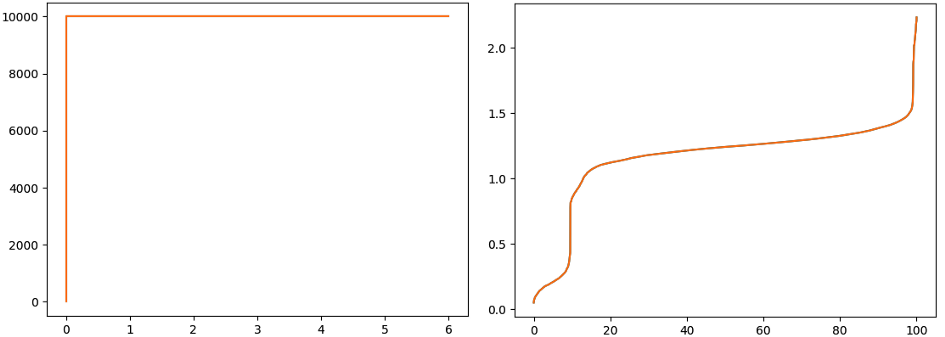
Throughput in RPS on the left, vs. overhead in ms from p0 to p100, on the right.
You can try to run the sync case with 10k RPS and see how such a rate is handled, and how blocking I/O scales, how many threads you need, and how much memory it requires:
concurrency-demo-benchmarks \
--name sync_10k_20ms \
--rate 10000 \
--num_req 100000 \
--latency "20ms*10" \
sync --threads $find_out
Is there any reason to use blocking I/O?
Writing applications using non-blocking I/O used to be non-trivial, either using the epoll system, complex state machines, or dealing with so-called callback hell. The concept of futures/promises made async programming much more accessible. However, it may still quickly get out of hand with chaining futures, handling failures/timeouts, and making mistakes, such as inserting long-running operations into I/O threads.
Most major languages support async/await syntax, allowing writing, reading, and maintaining asynchronous routines to be almost as simple as blocking synchronous code.
For instance, in my post about writing an HTTP Tunnel in Rust, I tried to demonstrate the straightforwardness of building a performant and fault-tolerant network service using async I/O.\
Translating to intuitive terms of the road-system analogy:
Blocking I/O —a very wide (and thus expensive) freeway. This is great until a car gets stalled, or there is a slowdown, causing a miles-long traffic jam.
Async I/O — a freeway with just a few lanes and a vast parking lot for cars that failed or are waiting, so the road never gets congested. I wish all streets were like this, but at least we can build such services.
Source: Medium
The Tech Platform
Comments