Data Scientist’s Guide to Efficient Coding in Python
- The Tech Platform
- Oct 22, 2021
- 8 min read
We are giving real-life coding scenarios where we have actually used them!
1. Use tqdm when working with for loops.
Imagine looping over a large iterable (list, dictionary, tuple, set), and not knowing whether the code has finished running! Bummer, right! In such scenarios make sure to use tqdm construct to display a progress bar alongside.
For instance, to display the progress as I read through all the files present in 44 different directories (whose paths I have already stored in a list called fpaths):
from tqdm import tqdmfiles = list()
fpaths = ["dir1/subdir1", "dir2/subdir3", ......]
for fpath in tqdm(fpaths, desc="Looping over fpaths")):
files.extend(os.listdir(fpath))

Using tqdm with “for“ loop
Note: Use the desc argument to specify a small description for the loop.
2. Use type hinting when writing functions.
In simple terms, it means explicitly stating the type of all the arguments in your Python function definition.
I wish there were specific use cases I could provide to emphasize when I use type hinting for my work, but the truth is, I use them more often than not.
Here’s a hypothetical example of a function update_df(). It updates a given data frame by appending a row containing useful information from a simulation run — such as classifier used, accuracy scored, train-test split size, and additional remarks for that particular run.
def update_df(df: pd.DataFrame,
clf: str,
acc: float,
remarks: List[str] = []
split:float = 0.5) -> pd.DataFrame:
new_row = {'Classifier':clf,
'Accuracy':acc,
'split_size':split,
'Remarks':remarks}
df = df.append(new_row, ignore_index=True)
return df
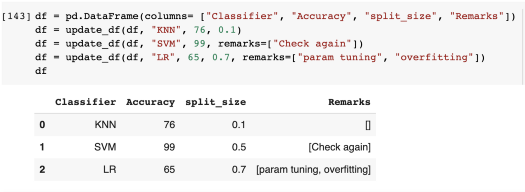
Few things to note:
The datatype following the -> symbol in the function definition (def update_df(.......) -> pd.DataFrame) indicates the type of the value returned by the functions, i.e. a Pandas’s dataframe in this case.
The default value, if any, can be specified as usual in the form param:type = value . (For example: split: float = 0.5)
In case a function does not return anything, feel free to use None. For example : def func(a: str, b: int) -> None: print(a,b)
To return values of mixed types, for example, say a function could either print a statement if a flag optionwas set OR return an intif the flag was not set:
from typing import Union
def dummy_args(*args: list[int], option = True) -> Union[None, int]:
if option:
print(args)
else:
return 10
Note: As of Python 3.10, Union is not required, so you can simply do:
def dummy_args(*args: list[int], option = True) -> None | int:
if option:
print(args)
else:
return 10
You can go as specific as you’d like while defining the types of parameters, as we have done for remarks: List[str]. Not only do we specify it should be a List, but it should be a list of str only. For fun, try passing a list of integers to remarkswhile calling the function. You’ll see no error returned! Why is that? Because Python interpreter doesn’t enforce any type checking based on your type-hints.
It’s still good practice, though, to include it! I feel it lends more clarity to oneself when writing a function. In addition, when someone makes a call to such a function, they get to see nice prompts for the arguments it would take as inputs.

Prompts when calling a function with type hinting
3. Use args and kwargs for functions with unknown # of arguments.
Imagine this: you want to write a function that takes as input some directory paths and prints the number of files within each. The problem is, we do not know how many paths the user would input! Could be 2 could be 20! So we are unsure how many parameters should we define in our function definition. Clearly, writing a function like def count_files(file1, file2, file3, …..file20)would be silly. In such cases, args and (sometimes kwargs) come handy!
Args is used for specifying an unknown number of positional arguments. Kwargs is used for specifying an unknown number of keyword arguments.
Args
Here’s an example of a function count_files_in_dir()that takes in project_root_dir and an arbitrary number of folder paths within it (using *fpaths in the function definition). As an output, it prints the number of files within each of these folders.
def count_files_in_dir(project_root_dir, *fpaths: str):
for path in fpaths:
rel_path = os.path.join(project_root_dir, path)
print(path, ":", len(os.listdir(rel_path)))
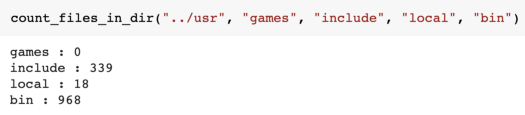
Counting the # of files in Google Colab directories
In the function call, we pass in 5 arguments. As the function definition expects one required positional arguments i.e. project_root_dir, it automatically knows "../usr" must be it. All the remaining arguments (four in this case) are “soaked up” by *fpathsand are used for counting the files.
Note: The proper terminology for this soaking up technique is “argument packing” i.e. remaining arguments are packed into *fpaths.
Kwargs
Let’s look at functions that must take an unknown number of keyword arguments. In such scenarios, we must use kwargs instead of args. Here’s a short (useless) example:
def print_results(**results):
for key, val in results.items():
print(key, val)

The usage is quite similar to *args but now we are able to pass an arbitrary number of keyword arguments to a function. These arguments get stored as key-value pairs in **resultsdictionary. From hereon, it is easy to access the items within this dictionary using .items().
I have found two main applications for kwargs in my work:
merging dictionaries (useful but less interesting)
dict1 = {'a':2 , 'b': 20}
dict2 = {'c':15 , 'd': 40}
merged_dict = {**dict1, **dict2}
*************************
{'a': 2, 'b': 20, 'c': 15, 'd': 40}
extending an existing method (more interesting)
def myfunc(a, b, flag, **kwargs):
if flag:
a, b = do_some_computation(a,b)
actual_function(a,b, **kwargs)
Here’s a real practical use-case for extending methods using **kwargs from one of my recent projects:
We often use Sklearn’s train_test_split() for splitting X and y. While working on one of the GANs projects, I had to split the generated synthetic images into the same train-test split that is used for splitting real images and their respective labels. In addition, I also wanted to be able to pass any other arguments that one would normally pass to the train_test_split(). Finally, stratify must always be passed because I was working on a face recognition problem (and wanted all labels to be present in both train and test set).
To achieve this, we created a function called custom_train_test_split(). I’ve included a bunch of print statements to show what exactly is happening under the hood (and omitted some snippets for brevity).
def custom_train_test_split(clf, y, *X, stratify, **split_args):
print("Classifier used: ", classifier)
print("Keys:", split_args.keys())
print("Values: ", split_args.values())
print(X)
print(y)
print("Length of passed keyword arguments: ", len(split_args))
trainx,testx,*synthetic,trainy,testy = train_test_split(
*X,
y,
stratify=stratify,
**split_args
)
######### OMITTED CODE SNIPPET #############
# Train classifier on train and synthetic ims
# Calculate accuracy on testx, testy ############################################
print("trainx: ", trainx, "trainy: ",trainy, '\n', "testx: ", testx, "testy:", testy)
print("synthetic: ", *synthetic)
Note: While calling this function, instead of using the actual image vectors and labels (see figure below), I have replaced them with dummy data for ease of understanding. The code, however, should work for real images as well!

Figure A Calling a function with kwargs in function definition
Bunch of things to note:
All the keyword arguments (except stratify), used in the function call statement (such as train_size and random_state) will be stored as a key-value pair in the **split_args dictionary. (To verify, check out the output in Blue.) Why not stratify, you might ask? This is because according to the function definition, it is a required keyword-only argument and not an optional one.
All the non-keyword (i.e. positional) arguments passed in the function call (such as "SVM", labels, etc.) would be stored in the first three parameters in function definition i.e.clf, y and *X, (and yes the order in which they are passed matters). However, we have four arguments in function call i.e. "SVM", labels, ims , and synthetic_ims. Where do we store the fourth one? Remember that we used *X as the third parameter in the function definition, hence all arguments passed to the function after the first two arguments are packed (soaked) into *X. (To verify, check output in Green).
When calling train_test_split() method within our function, we are essentially unpacking the X and split_args arguments using the * operator,(*X and **split_args), so that all elements of it can be passed as different parameters.
That is,
train_test_split(*X, y, stratify = stratify, **split_args)
is equivalent to writing
train_test_split(ims, synthetic_ims, y, stratify = stratify, train_size = 0.6, random_state = 50)
When storing the results from the train_test_split() method, we again pack the synthetic_train and synthetic_test sets into a single *synthetic variable.
To check what’s inside it, we can unpack it again using * operator (see the output in Pink).
4. Use pre-commit hooks.
The code we write is often messy and lacks proper formatting such as trailing whitespaces, trailing commas, unsorted import statements, spaces in indentation, etc.
While it is possible to fix all this manually, you will be saving yourself a great deal of time with pre-commit hooks. In simpler terms, these hooks can perform auto-formatting for you with one line of command — pre-commit run.
Here are some simple steps from the official documentation to get started and create a .pre-commit-config.yaml file. It will contain hooks for all the formatting issues that you care about!
As a purely personal preference, I tend to keep my .pre-commit-config.yaml configuration file simple and stick with Black’s pre-commit configuration.
Note: One thing to remember is that files must be staged i.e. git add . before you do pre-commit run , otherwise, you’ll see all the files will be Skipped :
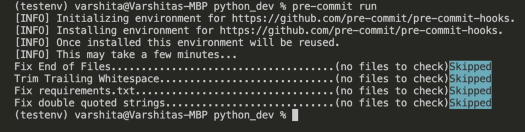
5. Use .yml config files to store constants.
If your project contains a lot of config variables such as database hostname, passwords, AWS credentials, etc. use a .yml file to keep track of all of them. You can later use this file in any Jupyter Notebook or script that you wish.
Given most of my work is aimed at providing a model framework to clients so they can train it again on their own dataset, I usually use config files for storing paths to folders and files. It is also a nice way to make sure the client has to make changes to only one file when running your script at their end.
Let’s go ahead and create a fpaths.yml file in the project directory. We will be storing the root directory where images must be stored. Additionally, the paths to the file names, labels, attributes, etc. Finally, we also store the paths to synthetic images.
image_data_dir: path/to/img/dir
# the following paths are relative to images_data_dir
fnames:
fnames_fname: fnames.txt
fnames_label: labels.txt
fnames_attr: attr.txt
synthetic:
edit_method: interface_edits
expression: smile.pkl
pose: pose.pkl
You can read this file like:
# open the yml file
with open(CONFIG_FPATH) as f:
dictionary = yaml.safe_load(f)
# print elements in dictionary
for key, value in dictionary.items():
print(key + " : " + str(value))
print()
Note: If you’d like to dig deeper, here’s a brilliant tutorial to get you started with yaml.
6. Bonus: Useful VS-Code Extensions.
While there are certainly many nice choices for Python editors, I must say VSCode is hands-down the best I have seen so far (sorry, Pycharm). To get even more use of it, consider installing these extensions from the marketplace:
Bracket Pair Colorizer — allows matching brackets to be identified with colors.

Path Intellisense — allows autocompleting filenames.
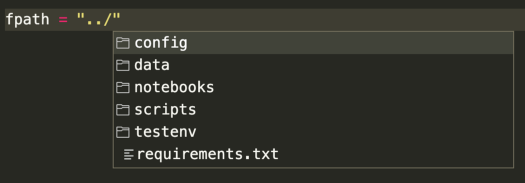
Python Dockstring generator — allows generating docstrings for Python functions.

Generating docstrings using VScode extension
Pro-tip: Generate the docstring (using """) after you’ve written the function using type hinting. This way, the docstring generated will be even richer in information such as default value, argument types, etc. (See image on right above).
Python Indent — (my favorite; published by by Kevin Rose) allows proper indentation of code/brackets that runs on multiple lines.
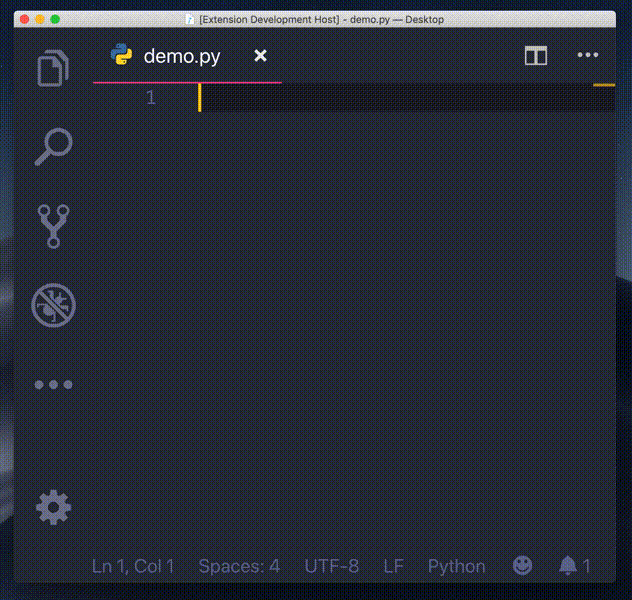
Source: VSCode Extensions Marketplace
Python Type Hint — allows auto-completion for type hints when writing functions.

TODO tree: (second favorite) keeps track of ALL the TODO's at one place that were inserted while writing scripts.
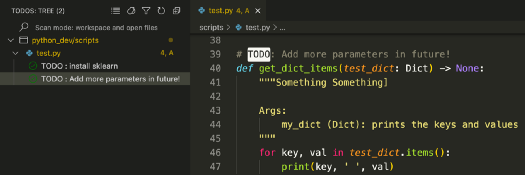
Keep track of all TODO comments inserted in your project
Pylance — allows code completion, parameter suggestions (and a loootttt more for writing code faster).
Congrats on being one step closer to a professional Python developer. I intend to keep updating this article as and when I learn more cool tricks. As always, if there’s an easier way to do some of the things I mentioned in this article, please do let me know.
Source: KDNuggets
The Tech Platform
Commenti